인공지능 영문 뉴스 (3)
A collaborative research team from the MIT-Takeda Program combined physics and machine learning to characterize rough particle surfaces in pharmaceutical pills and powders.
MIT-Takeda 프로그램의 공동 연구팀은 물리학과 기계 학습을 결합하여 약과 분말의 거친 입자 표면을 특성화했습니다.
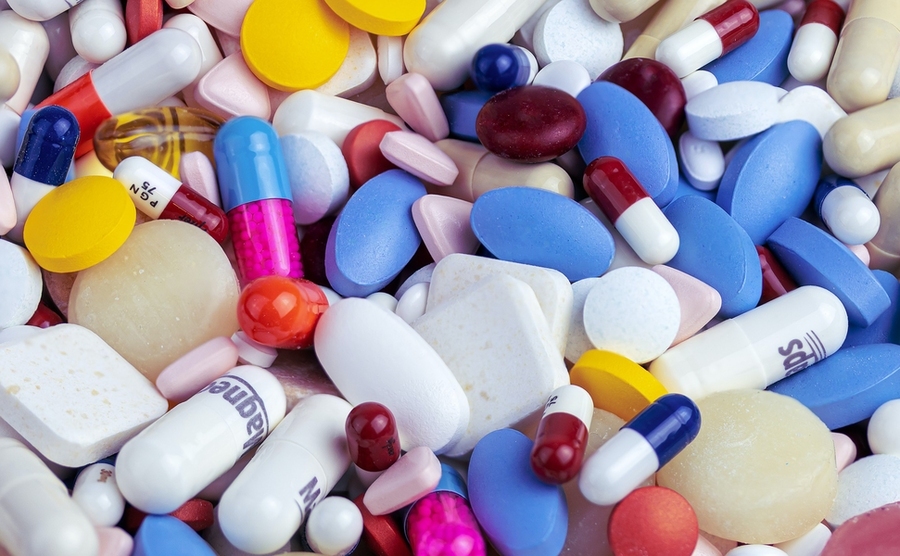
When medical companies manufacture the pills and tablets that treat any number of illnesses, aches, and pains, they need to isolate the active pharmaceutical ingredient from a suspension and dry it. The process requires a human operator to monitor an industrial dryer, agitate the material, and watch for the compound to take on the right qualities for compressing into medicine. The job depends heavily on the operator’s observations.
의료 회사들이 수많은 질병, 통증, 통증을 치료하는 알약과 정제를 제조할 때, 그들은 활성 의약품 성분을 현탁액으로부터 분리하고 건조시켜야 합니다. 이 과정에서 인간 작업자는 산업용 건조기를 모니터링하고, 재료를 휘젓고, 혼합물이 약으로 압축되는 데 적합한 품질을 띠는지 관찰해야 합니다. 작업은 작업자의 관측치에 크게 의존합니다.
Methods for making that process less subjective and a lot more efficient are the subject of a recent Nature Communications paper authored by researchers at MIT and Takeda. The paper’s authors devise a way to use physics and machine learning to categorize the rough surfaces that characterize particles in a mixture. The technique, which uses a physics-enhanced autocorrelation-based estimator (PEACE), could change pharmaceutical manufacturing processes for pills and powders, increasing efficiency and accuracy and resulting in fewer failed batches of pharmaceutical products.
그 과정을 덜 주관적이고 훨씬 더 효율적으로 만드는 방법은 MIT-Takeda의 연구원들이 최근 작성한 네이처 커뮤니케이션 논문의 주제입니다. 이 논문의 저자들은 혼합물의 입자를 특징짓는 거친 표면을 분류하기 위해 물리학과 기계 학습을 사용하는 방법을 고안합니다. 물리적으로 강화된 자기 상관 기반 추정기(PEACE)를 사용하는 이 기술은 알약과 분말에 대한 제약 제조 프로세스를 변경하여 효율성과 정확성을 높이고 실패한 의약품 배치를 줄일 수 있습니다.
“Failed batches or failed steps in the pharmaceutical process are very serious,” says Allan Myerson, a professor of practice in the MIT Department of Chemical Engineering and one of the study’s authors. “Anything that improves the reliability of the pharmaceutical manufacturing, reduces time, and improves compliance is a big deal.”
"제약 과정에서 실패한 배치나 실패한 단계는 매우 심각한 문제입니다," MIT 화학 공학부의 실습 교수이자 이 연구의 저자 중 한 명인 앨런 마이어슨이 말합니다. "제약 제조의 신뢰성을 높이고, 시간을 단축하며, 규정 준수를 개선하는 것은 무엇이든 중요합니다."
The team’s work is part of an ongoing collaboration between Takeda and MIT, launched in 2020. The MIT-Takeda Program aims to leverage the experience of both MIT and Takeda to solve problems at the intersection of medicine, artificial intelligence, and health care.
이 팀의 작업은 2020년에 시작된 Takeda와 MIT 간의 진행 중인 협업의 일부입니다. MIT-Takeda 프로그램은 MIT와 Takeda의 경험을 활용하여 의학, 인공지능 및 건강 관리의 교차점에서 문제를 해결하는 것을 목표로 합니다.
In pharmaceutical manufacturing, determining whether a compound is adequately mixed and dried ordinarily requires stopping an industrial-sized dryer and taking samples off the manufacturing line for testing. Researchers at Takeda thought artificial intelligence could improve the task and reduce stoppages that slow down production. Originally the research team planned to use videos to train a computer model to replace a human operator. But determining which videos to use to train the model still proved too subjective. Instead, the MIT-Takeda team decided to illuminate particles with a laser during filtration and drying, and measure particle size distribution using physics and machine learning.
제약 제조에서 화합물이 적절하게 혼합되고 건조되는지 여부를 결정하려면 일반적으로 산업용 크기의 건조기를 중지하고 테스트를 위해 제조 라인에서 샘플을 추출해야 합니다. Takeda의 연구원들은 인공지능이 작업을 개선하고 생산을 늦추는 중단 현상을 줄일 수 있다고 생각했습니다. 원래 그 연구팀은 인간 운영자를 대체할 컴퓨터 모델을 훈련시키기 위해 비디오를 사용할 계획이었습니다. 그러나 모델을 교육하는 데 사용할 비디오를 결정하는 것은 여전히 너무 주관적인 것으로 판명되었습니다. 대신 MIT-Takeda 팀은 여과와 건조 과정에서 입자를 레이저로 비추고 물리학과 기계 학습을 이용해 입자 크기 분포를 측정하기로 결정했습니다.
“We just shine a laser beam on top of this drying surface and observe,” says Qihang Zhang, a doctoral student in MIT’s Department of Electrical Engineering and Computer Science and the study’s first author.
"우리는 이 건조한 표면 위에 레이저 광선을 비추고 관찰할 뿐입니다," MIT 전기 공학 및 컴퓨터 과학부의 박사 과정 학생이자 이 연구의 첫 번째 저자인 Qihang Zhang이 말합니다.
A physics-derived equation describes the interaction between the laser and the mixture, while machine learning characterizes the particle sizes. The process doesn’t require stopping and starting the process, which means the entire job is more secure and more efficient than standard operating procedure, according to George Barbastathis, professor of mechanical engineering at MIT and corresponding author of the study.
물리학에서 파생된 방정식은 레이저와 혼합물 사이의 상호 작용을 설명하는 반면, 기계 학습은 입자 크기를 특징으로 합니다. MIT의 기계 공학 교수이자 이 연구의 상응하는 저자인 George Barbastathis에 따르면, 이는 전체 작업이 표준 작동 절차보다 더 안전하고 효율적이라는 것을 의미합니다.
The machine learning algorithm also does not require many datasets to learn its job, because the physics allows for speedy training of the neural network.
또한 기계 학습 알고리즘은 물리학이 신경망의 신속한 훈련을 허용하기 때문에 많은 데이터 세트를 학습할 필요가 없습니다.
“We utilize the physics to compensate for the lack of training data, so that we can train the neural network in an efficient way,” says Zhang. “Only a tiny amount of experimental data is enough to get a good result.”
"우리는 훈련 데이터의 부족을 보완하기 위해 물리학을 활용하여 신경망을 효율적인 방식으로 훈련할 수 있습니다."라고 Zhang은 말합니다. "아주 적은 양의 실험 데이터만으로도 좋은 결과를 얻을 수 있습니다."
Today, the only inline processes used for particle measurements in the pharmaceutical industry are for slurry products, where crystals float in a liquid. There is no method for measuring particles within a powder during mixing. Powders can be made from slurries, but when a liquid is filtered and dried its composition changes, requiring new measurements. In addition to making the process quicker and more efficient, using the PEACE mechanism makes the job safer because it requires less handling of potentially highly potent materials, the authors say.
오늘날 제약 산업에서 입자 측정에 사용되는 유일한 인라인 프로세스는 결정이 액체에 떠 있는 슬러리 제품입니다. 혼합 중에 분말 내의 입자를 측정하는 방법은 없습니다. 분말은 슬러리로 만들 수 있지만, 액체를 여과하고 건조하면 성분이 변하기 때문에 새로운 측정이 필요합니다. 공정을 더 빠르고 효율적으로 만드는 것 외에도, PEACE 메커니즘을 사용하면 잠재적으로 매우 강력한 물질의 취급이 덜 필요하기 때문에 작업이 더 안전하다고 저자들은 말합니다.
The ramifications for pharmaceutical manufacturing could be significant, allowing drug production to be more efficient, sustainable, and cost-effective, by reducing the number of experiments companies need to conduct when making products. Monitoring the characteristics of a drying mixture is an issue the industry has long struggled with, according to Charles Papageorgiou, the director of Takeda’s Process Chemistry Development group and one of the study’s authors.
제약 제조에 미치는 영향은 상당할 수 있으며, 기업이 제품을 제조할 때 수행해야 하는 실험 수를 줄임으로써 의약품 생산이 보다 효율적이고 지속 가능하며 비용 효율적일 수 있습니다. Takeda 공정 화학 개발 그룹의 책임자이자 이 연구의 저자 중 한 명인 Charles Papageorgiou에 따르면 건조 혼합물의 특성을 모니터링하는 것은 업계가 오랫동안 씨름해온 문제입니다.
“It is a problem that a lot of people are trying to solve, and there isn’t a good sensor out there,” says Papageorgiou. “This is a pretty big step change, I think, with respect to being able to monitor, in real time, particle size distribution.”
Papageorgiou는 "많은 사람들이 해결하려고 노력하는 문제이며, 밖에는 좋은 센서가 없습니다,"라고 말합니다. "이것은 입자 크기 분포를 실시간으로 모니터링할 수 있다는 점에서 상당히 큰 단계 변화라고 생각합니다."
Papageorgiou said that the mechanism could have applications in other industrial pharmaceutical operations. At some point, the laser technology may be able to train video imaging, allowing manufacturers to use a camera for analysis rather than laser measurements. The company is now working to assess the tool on different compounds in its lab.
Papageorgiou는 이 메커니즘이 다른 산업 제약 작업에 적용될 수 있다고 말했습니다. 어느 시점에서 레이저 기술은 비디오 영상을 훈련시킬 수 있어 제조업체가 레이저 측정 대신 카메라를 분석에 사용할 수 있습니다. 이 회사는 현재 실험실의 여러 화합물에서 도구를 평가하기 위해 노력하고 있습니다.
The results come directly from collaboration between Takeda and three MIT departments: Mechanical Engineering, Chemical Engineering, and Electrical Engineering and Computer Science. Over the last three years, researchers at MIT and Takeda have worked together on 19 projects focused on applying machine learning and artificial intelligence to problems in the health-care and medical industry as part of the MIT-Takeda Program.
이 결과는 Takeda와 세 개의 MIT 부서 간의 협업에서 얻어졌습니다: 기계 공학, 화학 공학, 그리고 전기 공학과 컴퓨터 과학. MIT와 Takeda의 연구원들은 지난 3년 동안 MIT-Takeda 프로그램의 일환으로 의료 및 의료 산업의 문제에 기계 학습과 인공 지능을 적용하는 데 초점을 맞춘 19개 프로젝트에 함께 일해 왔습니다.
Often, it can take years for academic research to translate to industrial processes. But researchers are hopeful that direct collaboration could shorten that timeline. Takeda is a walking distance away from MIT’s campus, which allowed researchers to set up tests in the company’s lab, and real-time feedback from Takeda helped MIT researchers structure their research based on the company’s equipment and operations.
종종, 학술 연구가 산업 과정으로 전환되는 데 몇 년이 걸릴 수 있습니다. 하지만 연구원들은 직접적인 협력이 그 일정을 단축시킬 수 있을 것으로 기대하고 있습니다. Takeda는 MIT 캠퍼스에서 걸어갈 수 있는 거리로 연구원들이 회사 연구실에서 테스트를 진행할 수 있었고, Takeda의 실시간 피드백은 MIT 연구원들이 회사의 장비와 운영을 기반으로 연구를 구성하는 데 도움이 되었습니다.
Combining the expertise and mission of both entities helps researchers ensure their experimental results will have real-world implications. The team has already filed for two patents and has plans to file for a third.
두 기관의 전문성과 임무를 결합하면 연구자들이 실험 결과가 실제 세계에 영향을 미칠 것임을 보장하는 데 도움이 됩니다. 그 팀은 이미 두 개의 특허를 신청했고 세 번째 특허를 신청할 계획입니다.
댓글